Publications
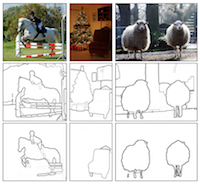
K.K. Maninis,
J. Pont-Tuset,
P. Arbeláez, and
L. Van Gool
Convolutional Oriented Boundaries: From Image Segmentation to High-Level Tasks
IEEE Transactions on Pattern Analysis and Machine Intelligence (TPAMI), vol. 40, no. 4, pp. 819 - 833, 2018.
[PDF] [BibTex]
@article{Man+18a,
author = {Kevis-Kokitsi Maninis and Jordi Pont-Tuset and Pablo Arbel\'{a}ez and Luc Van Gool},
title = {Convolutional Oriented Boundaries: From Image Segmentation to High-Level Tasks},
journal = {IEEE Transactions on Pattern Analysis and Machine Intelligence (TPAMI)},
year = {2018},
volume = {40},
number = {4},
pages = {819 - 833}
}
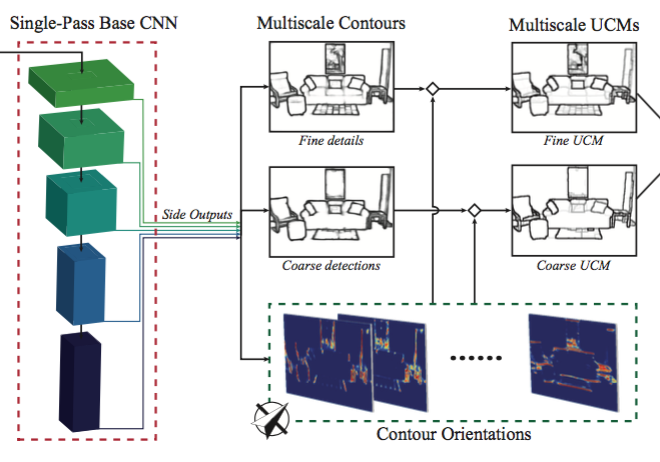
K.K. Maninis,
J. Pont-Tuset,
P. Arbeláez, and
L. Van Gool
Convolutional Oriented Boundaries
European Conference on Computer Vision (ECCV) 2016
[PDF] [BibTex]
@inproceedings{Man+16a,
author = {Kevis-Kokitsi Maninis and Jordi Pont-Tuset and Pablo Arbel\'{a}ez and Luc Van Gool},
title = {Convolutional Oriented Boundaries},
booktitle = {European Conference on Computer Vision (ECCV)},
year = {2016}
}
Abstract
This paper presents Convolutional Oriented Boundaries (COB), which produces multiscale oriented contours and region hierarchies starting from generic image classification CNNs. COB is computationally efficient, because it requires a single CNN forward pass for contour detection and uses a novel sparse boundary representation for hierarchical segmentation; it gives a significant leap in performance over the state-of-the-art, and it generalizes very well to unseen categories and datasets. We perform extensive experiments on BSDS, PASCAL Context, PASCAL Segmentation, and MS-COCO, showing that COB provides state-of-the-art contours, region hierarchies, and object proposals.
Demo of the Detected Orientations, UCM, and Object Proposal
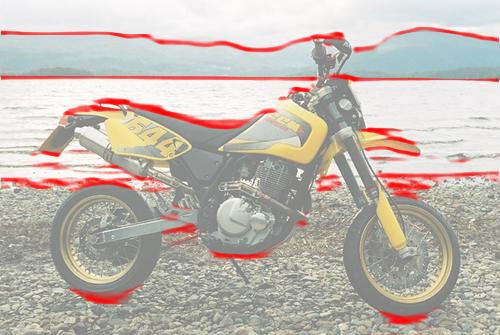
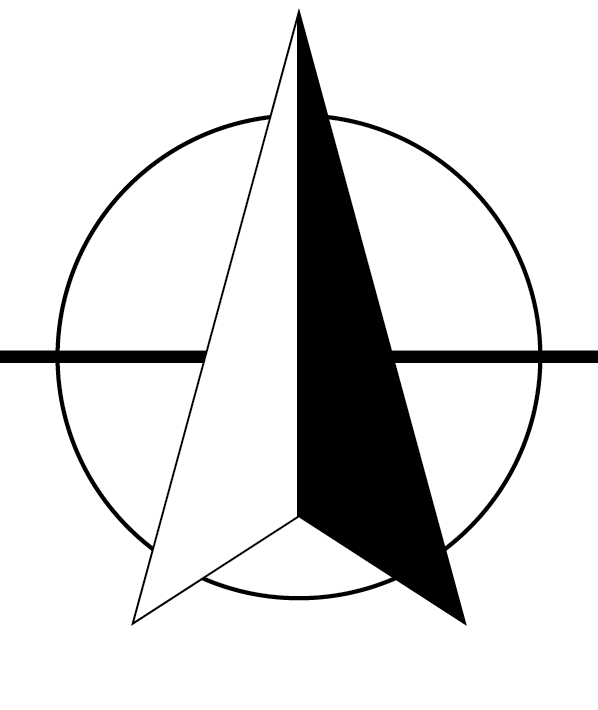
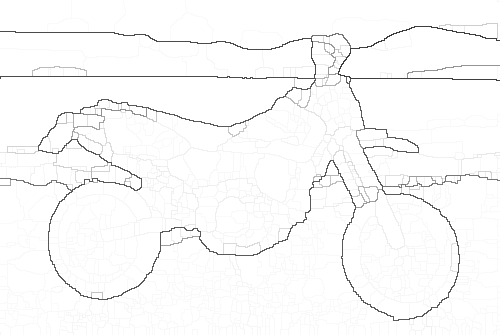
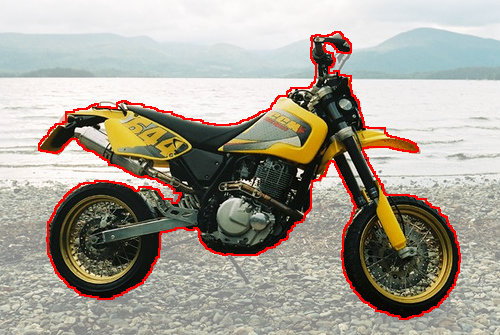
Benchmark State of the Art
Display the quantitative evaluation of the current State-of-the-Art techniques in PASCAL Context.
Explore State of the Art
Qualitatively compare current State-of-the-Art techniques in PASCAL Context.
Code & Pre-Computed Results
Download the COB code and pre-computed COB segmentations and proposals in a variety of popular datasets: BSDS500, MS-COCO, PASCAL, etc.